Anonymous Co-op Interview Selection at CharityCAN
Anonymous Hiring
Back in the summer of 2020, with Black Lives Matter marches happening in every major city in North America, I was challenged to take a look at the diversity, equity and inclusion (DEI) practices at our little company. As anyone who works at a small business can attest to, there aren’t often official practices or procedures put in place until something goes wrong and forces you to create something to adhere to.
As a company that straddles the fundraising and software industries, we’re in a double whammy of fields that are predominantly white (in the case of the former) and predominantly white and male (in the latter). Our current staff is split 50/50 between genders, but we only have one team member who is racialized (or 12% of the company).
With that in mind, I contacted Lunaria, a local company that helps companies with their DEI practices. While taking me through some things to consider, Lunaria suggested hiring practices as one place a small company could look to reduce unconscious bias and make sure we’re finding the best candidates regardless of race or gender. While we don’t have any open job positions on the immediate horizon, we do hire a co-op student every four months to help on the software development team. I wasn’t sure how we would do it, but this term I made it a goal to use anonymous hiring, or blind recruitment – stripping away any identifiable information from job applications to reduce bias – while selecting co-op students to interview.
The Baseline
To start out, I was curious to see if I could pull together information about the co-op students from the University of Waterloo (UW) (where we hire our co-op students from). This would let me see whether or not we were attracting and selecting students that were more or less in line with the race and gender of the overall student body.
Using the Common University Data Set from UW , I was able to get a breakdown of students along gender lines from the programs we hire our co-ops from, Engineering and Computer Science (CS):
Gender | Engineering | Computer Science | Both Programs |
Male | 5,428 (71%) | 2,296 (75%) | 7,724 (72%) |
Female | 2,220 (29%) | 767 (25%) | 2,987 (28%) |
Race-based data was much more difficult to come by. It turns out that most Canadian universities do not collect race-based data, despite calls that say race-based data is necessary in order to address inequality and support students.
The closest thing I could track down was a demographic survey on the /r/uWaterloo subreddit. Despite all of the obvious issues with a self-reported survey from a small internet community, let’s take a look at the reported racial breakdown in the Math and Engineering faculties (note that this is slightly different than the Engineering/CS breakdown above – CS is only one part of the Math faculty at UW, but it still gives us some idea). The survey creators also posted a breakdown of the subreddit’s reported race vs. Ontario demographics in general, if that helps give some idea of who may be under/over-reported in the results.
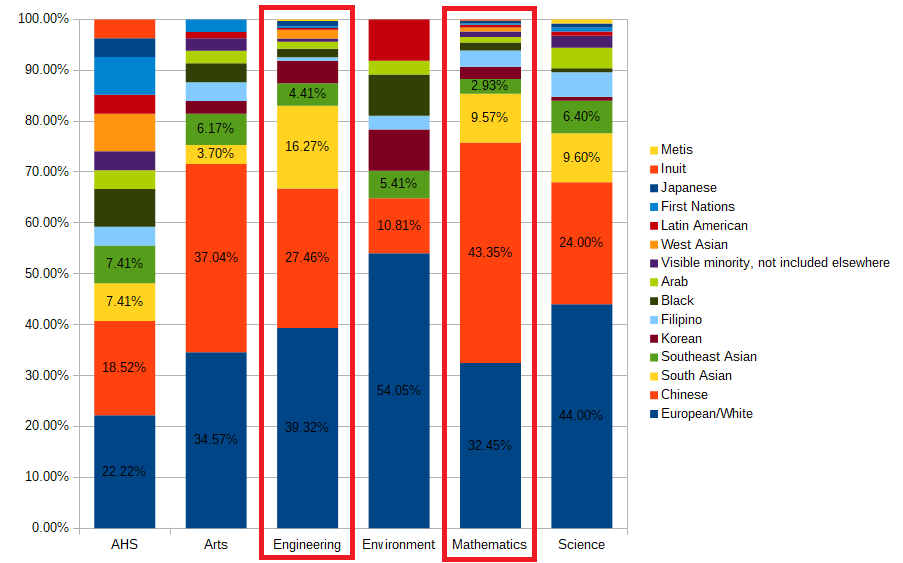
The Anonymous Selection Process
Now let’s take a look at our hiring process! Every four month term, we submit a job posting to the University of Waterloo’s co-op job portal, Waterloo Works. Students who are interested in the position post a PDF copy of their resume, and the university includes a student grade report and past co-op employer evaluations. I review these application packages and select students for the next stage, the in-person interview.
Following another of Lunaria’s suggestions, I asked our current UW co-op to help with the anonymization experiment, and they took the time to black out identifying student information in the applications (names, addresses, emails, etc.), leaving only their student numbers behind for reference.
Next came the selection process. I was surprised at how much my old (non-anonymous) process relied on names until they were gone! My brain was apparently trained to use a student’s name as a placeholder in my head, and with student numbers any sort of personality I might have built up completely disappeared. It made remembering which resumes I had already read a little difficult, but it’s easy to see how unconscious bias seeps in without you even thinking about it.
Something else I noticed was that it’s probably not enough just to scrub names and email addresses – next time I’ll probably scrub any “interests” from the resumes as well. They made it too easy to make a gender (e.g. “mixed martial arts” vs. “figure skating”) or race (“Chinese Student Association” vs. “Minor League Hockey Referee”) assumption.
Other than that, it was no trouble to whittle down the resumes based on the anonymized data.
Results
Here are the results of the anonymous selection process broken down by gender and race (these are just guesses – the only way for me to identify student race and gender was to use names and LinkedIn profiles from applications). Out of 48 initial candidates, I selected 7 students for one-on-one interviews. Here’s how that looks:
Assumed Gender | # Students Applied | # Students Selected |
Male | 40 (83%) | 5 (71%) |
Female | 8 (17%) | 2 (29%) |
Assumed Race or Ethnic Background | # Students Applied | # Students Selected |
Asian | 24 (50%) | 1 (14%) |
Black | 1 (2%) | 1 (14%) |
Latinx | 1 (2%) | 0 (0%) |
Middle Eastern | 3 (6%) | 1 (14%) |
South Asian | 14 (29%) | 2 (29%) |
White | 5 (11%) | 2 (29%) |
So in the end I ended up with a fairly diverse group of students. If you look along gender lines, the anonymous process selected a number of candidates that matched up with the applicant pool.
But after all that I still ended up with an over-representation of white students! This is a small sample size, so maybe it doesn’t mean anything, but I wonder if some unconscious bias was still happening – through the interests section of the resumes, or the format of the resumes. Maybe the fact that these students are white means they have had better co-op jobs or were evaluated on those jobs better in the past? I also ended up with a severe under-representation of Asian students. Again, I’ll have to see if this is some sort of bias or whether we’re just looking at a small sample issue.
When I compare our applicant pool to the student body breakdown, it seems like our applicants are more or less as racially diverse as the general student population, but it doesn’t look like we’re attracting quite as many female candidates as are in the general student population. We did end up with a pretty good representation in our selected candidates, however. Maybe we can update our future job descriptions to make them more inclusive.
In the end, the anonymous selection added negligible overhead and seems to have worked out so far! I’m looking forward to using it again next term and finding other ways to improve our process.